[video] Martin Riedmiller - 2019-03-29
DeepMind
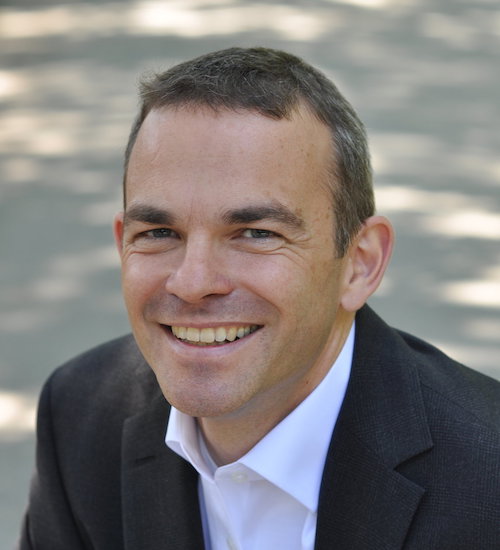
Title
Machines that learn from scratch
Abstract
Being able to autonomously learn ‘from scratch’—i.e. with a minimum amount of prior knowledge—is a key ability of intelligent systems. This credo is the driving motivation behind our research on reinforcement learning methods for the control of dynamical systems. While we have seen tremendous progress in the area of deep reinforcement learning in the last couple of years, its direct application to real systems still remains a challenge. Key requirements for agents mastering the real world are data-efficiency and reliability of learning, since data-collection in real environments, e.g. on real robots, is time intensive and often expensive.
I will highlight two main areas of progress that we consider crucial for progress towards this goal—improved off-policy learning methods from large data sets and better exploration. I will give examples of simulated and real robots that, by following these principles, can learn increasingly complex tasks from scratch.
Registration